Predicting the Next Technological Leap in AI Nude Filters for Adult Content
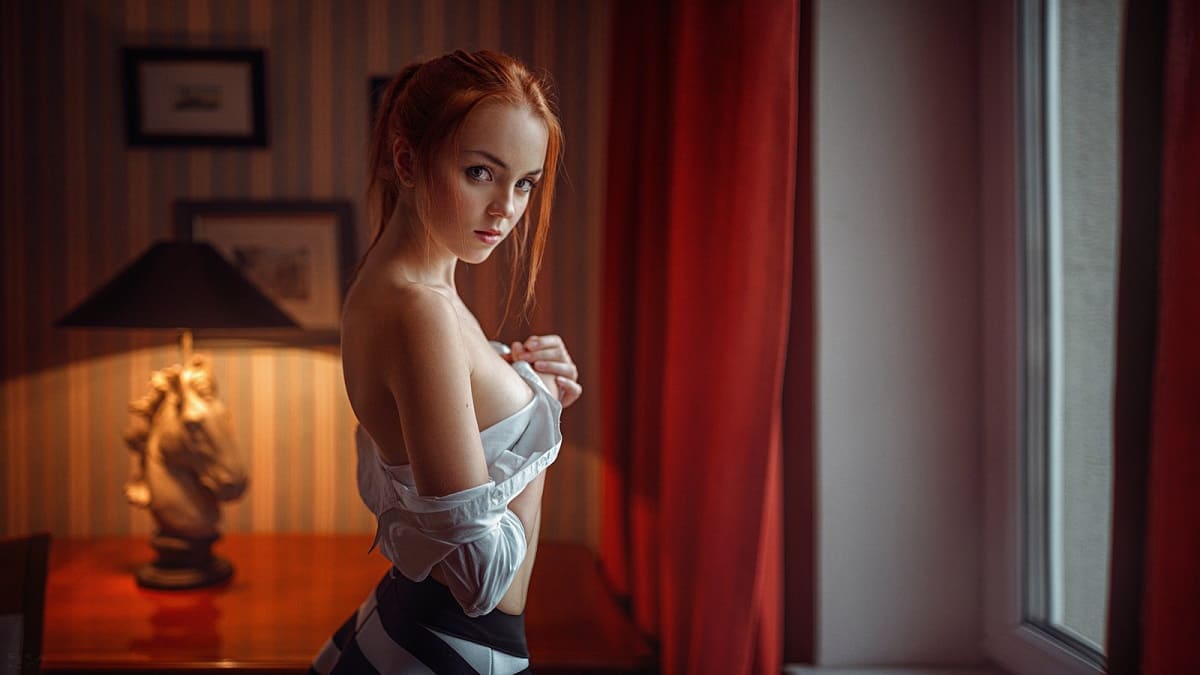
Predicting the next technological leap in AI nude filters for adult content involves anticipating advancements that will further enhance realism, customization, and interactivity. Future developments may include more sophisticated algorithms, improved integration with virtual and augmented reality, and greater personalization capabilities. These advancements will likely set new standards for user experience and content quality, driving innovation and growth in the industry. Staying informed about potential technological leaps is crucial for leveraging new opportunities and maintaining a competitive edge.
Current State of AI Nude Filters
AI nude filters have made significant strides in recent years, revolutionizing content moderation and privacy in digital platforms. These filters employ sophisticated algorithms to analyze images and videos, detecting nudity and explicit content with varying degrees of accuracy. Platforms like Facebook and Instagram utilize AI to automatically flag and remove inappropriate content, ensuring user safety and compliance with community guidelines.
Despite advancements, challenges persist, including false positives and the evasion tactics employed by content creators. Improving these filters involves refining AI models, enhancing training datasets, and addressing ethical concerns surrounding user privacy and algorithmic bias. As AI technology continues to evolve, the landscape of nude content moderation is poised for further innovation and refinement.
Challenges with Current AI Solutions
Current AI solutions for nude content detection face several key challenges that hinder their effectiveness and adoption:
- Accuracy Issues:
- High rates of false positives and false negatives.
- Difficulty in accurately discerning context and artistic nudity from explicit content.
- Privacy Concerns:
- Potential breaches of user privacy during content scanning.
- Risks associated with storing and processing sensitive images.
- Algorithmic Bias:
- Bias in training data leading to skewed results, impacting marginalized groups disproportionately.
- Challenges in developing inclusive and unbiased AI models.
- Evasion Tactics:
- Techniques used by content creators to circumvent detection algorithms.
- Rapid adaptation to new detection methods by malicious actors.
Addressing these challenges requires ongoing research and development in AI, machine learning, and ethical frameworks to ensure responsible deployment and enhancement of nude content detection technologies.
Emerging AI Technologies in Image Recognition
Emerging AI technologies in image recognition are poised to revolutionize the field of nude content detection. Recent advancements in deep learning, particularly in convolutional neural networks (CNNs) and recurrent neural networks (RNNs), have significantly enhanced the accuracy and efficiency of image analysis tasks. These technologies enable AI systems to learn intricate patterns and features within images, improving their ability to detect nudity with higher precision.
Moreover, developments in transfer learning and federated learning are expanding the capabilities of AI models in image recognition. Transfer learning allows AI systems to leverage knowledge from one task or domain to enhance performance in another, facilitating faster training and adaptation of nude content detection models. Federated learning, on the other hand, enables collaborative training of AI models across decentralized data sources while preserving data privacy—a critical consideration in sensitive applications like nude content detection.
As these technologies continue to evolve, they promise to address current limitations in AI nude filters by enhancing accuracy, scalability, and privacy preservation. The future integration of these advancements could lead to more robust and reliable solutions for content moderation in digital platforms.
Machine Learning and Deep Learning Innovations
Advancements |
Description |
Applications |
Convolutional Neural Networks (CNNs) |
Specialized for image analysis, capable of learning hierarchical features. |
Image recognition, object detection, nude content detection. |
Recurrent Neural Networks (RNNs) |
Process sequential data, useful for understanding context in images and videos. |
Video analysis, natural language processing (NLP). |
Transfer Learning |
Utilizes pre-trained models for faster adaptation to new tasks and datasets. |
Rapid deployment of AI models, improved accuracy in niche domains. |
Federated Learning |
Collaborative learning without centralized data storage, preserves user privacy. |
Healthcare AI, personalized recommendations, decentralized training. |
- CNNs are pivotal in detecting subtle patterns indicative of nudity, improving accuracy in distinguishing explicit content from benign images.
- RNNs enable AI systems to analyze video sequences, enhancing real-time detection capabilities and contextual understanding.
- Transfer learning accelerates model development by leveraging existing knowledge, facilitating quicker deployment and customization for specific content moderation needs.
- Federated learning addresses privacy concerns by training AI models on distributed data sources, ensuring sensitive image data remains secure and compliant with privacy regulations.
Machine learning and deep learning innovations continue to drive advancements in AI nude filters, promising more robust solutions for content moderation in digital platforms. These technologies not only improve the accuracy and efficiency of detecting nudity but also enhance privacy protections and adaptability to evolving content creation techniques. As research and development in AI continue to evolve, the integration of these innovations holds the potential to reshape the landscape of digital content moderation, ensuring safer and more secure online environments for users worldwide.
Impact of Generative Adversarial Networks (GANs)
Generative Adversarial Networks (GANs) have profoundly influenced the field of image generation and analysis, including applications in nude content detection:
- Image Generation and Realism:
- GANs are capable of generating highly realistic images, including nude content, indistinguishable from real photographs.
- This realism poses challenges for AI nude filters, as detecting artificially generated explicit content becomes increasingly difficult.
- Adversarial Training:
- GANs can be used to train AI models to improve their ability to detect subtle nuances and variations in nude images.
- Adversarial training enhances the robustness of AI nude filters against sophisticated evasion tactics employed by content creators.
- Ethical Considerations:
- The use of GANs in generating and detecting explicit content raises ethical concerns regarding privacy and consent.
- Addressing these concerns is crucial to ensuring responsible deployment of AI technologies in sensitive domains like nude content detection.
- Technological Arms Race:
- The advancement of GAN technology fuels a technological arms race between content creators and AI developers.
- This competition drives continuous innovation in both offensive (content creation) and defensive (content detection) AI applications.
As GAN technology continues to evolve, its impact on AI nude filters will likely shape future developments in content moderation and digital ethics. Striking a balance between technological advancement and ethical considerations remains paramount in leveraging GANs for responsible innovation.
Integration of Blockchain for Privacy and Security
Blockchain technology offers innovative solutions to enhance privacy and security in AI nude filters:
Privacy Enhancement:
- Decentralized Storage: Utilizing blockchain for decentralized storage of sensitive data, ensuring user anonymity and reducing the risk of data breaches.
- Immutable Records: Immutable records on the blockchain provide transparency and accountability in data handling practices, enhancing user trust.
Security Measures:
- Smart Contracts: Implementing smart contracts to enforce privacy policies and data access permissions without relying on centralized authorities.
- Tamper-Proof Data: Utilizing blockchain’s cryptographic techniques to secure data integrity and prevent unauthorized alterations.
Challenges and Considerations:
- Scalability: Addressing scalability issues to accommodate the large volume of image data processed by AI filters.
- Regulatory Compliance: Navigating regulatory frameworks to ensure compliance with data protection laws and privacy regulations.
Integrating blockchain technology into AI nude filters holds promise for improving data privacy, enhancing security, and fostering greater trust in digital content moderation practices. As blockchain continues to evolve, its applications in safeguarding sensitive information are poised to play a crucial role in shaping the future of AI-driven content moderation.